Document Type
Article
Publication Date
6-1-2023
Publication Title
Neuroimage: Reports
Publication Title
Neuroimage: Reports
Volume
3
Issue
2
Abstract
Efforts to predict trait phenotypes based on functional MRI data from large cohorts have been hampered by low prediction accuracy and/or small effect sizes. Although these findings are highly replicable, the small effect sizes are somewhat surprising given the presumed brain basis of phenotypic traits such as neuroticism and fluid intelligence. We aim to replicate previous work and additionally test multiple data manipulations that may improve prediction accuracy by addressing data pollution challenges. Specifically, we added additional fMRI features, averaged the target phenotype across multiple measurements to obtain more accurate estimates of the underlying trait, balanced the target phenotype's distribution through undersampling of majority scores, and identified data-driven subtypes to investigate the impact of between-participant heterogeneity. Our results replicated prior results from Dadi et al. (2021) in a larger sample. Each data manipulation further led to small but consistent improvements in prediction accuracy, which were largely additive when combining multiple data manipulations. Combining data manipulations (i.e., extended fMRI features, averaged target phenotype, balanced target phenotype distribution) led to a three-fold increase in prediction accuracy for fluid intelligence compared to prior work. These findings highlight the benefit of several relatively easy and low-cost data manipulations, which may positively impact future work.
Recommended Citation
Easley, Ty; Chen, Ruiqi; Hannon, Kayla; Dutt, Rosie; and Bijsterbosch, Janine, "Population Modeling with Machine Learning can Enhance Measures of Mental Health - Open-Data Replication" (2023). Statistical and Data Sciences: Faculty Publications, Smith College, Northampton, MA.
https://scholarworks.smith.edu/sds_facpubs/48
Digital Object Identifier (DOI)
10.1016/j.ynirp.2023.100163
Rights
© 2023 Published by Elsevier Inc.
Creative Commons License
This work is licensed under a Creative Commons Attribution-NonCommercial-No Derivative Works 4.0 International License.
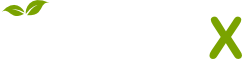
- Citations
- Citation Indexes: 2
- Usage
- Downloads: 95
- Abstract Views: 17
- Captures
- Readers: 46
- Mentions
- News Mentions: 1
Comments
Archived as published.